Philly Researchers Are Using the Latest in AI to Improve Cancer Care
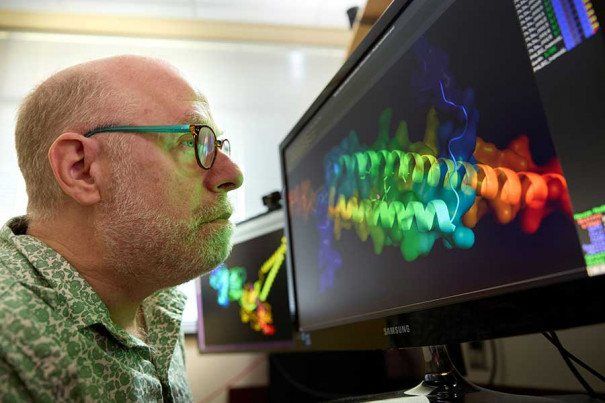
Courtesy of Fox Chase Cancer Center
You’ve heard of speech recognition and robotics, but you likely haven’t heard of one of the most significant AI achievements to date: AlphaFold. A software platform from Google’s Deep Mind, AlphaFold is revolutionizing how we understand biology, starting with accurately predicting protein structures.
DeepMind made AlphaFold2 available in July, and Fox Chase Cancer Center in Philadelphia quickly became one of the first medical centers in the country to put the new technology to widespread use. According to Fox Chase Researcher, Roland L. Dunbrack, Jr., PhD, this technology has major implications for drug development and healthcare in general.
“It just changes the game significantly,” Dunbrack says.
Proteins were a natural choice for DeepMind to focus on because they’re involved in every important activity that happens in the human body, from enabling muscles to contract to digesting food. What a protein does and how it works, however, is largely dependent on its unique 3D structure. Scientists have spent decades figuring out how proteins fold into particular shapes, to the point where the challenge has been dubbed the “protein folding problem.” AlphaFold’s ability to predict a protein’s shape has huge ramifications in many areas of biology, namely in allowing us to develop more targeted drugs — and develop them more quickly.
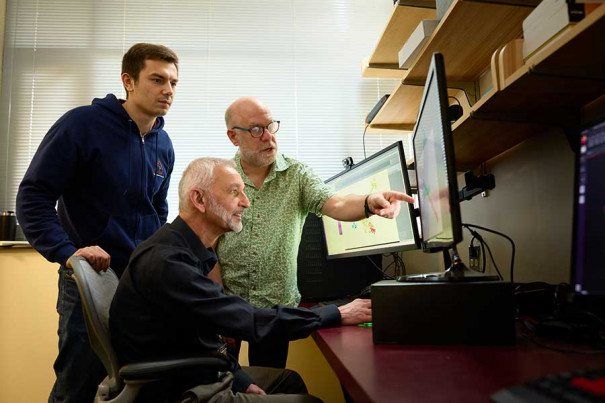
Courtesy of Fox Chase Cancer Center
Dunbrack is the director of the Molecular Modeling Facility at Fox Chase. His team works with researchers and physicians throughout the medical center, providing protein structure predictions and analysis, which help them learn more about issues such as mutations that arise in cancer or the best drugs to treat a given illness. They also sit down with the faculty member, scientist or graduate student who requested the prediction to explain the significance of the findings, and, if a paper comes out of the findings, will pull together graphics and descriptions of the protein structures.
“We don’t do the structure prediction and just hand it to the faculty member who requested it, because protein structures aren’t necessarily something they’re used to looking at and thinking about,” Dunbrack says. “Whereas I’ve been looking at structures for 30 years, and so I can look at it and predict whether a mutation would be debilitating. We not only do the structure prediction, but we bring our expertise and experience to clinicians and researchers at Fox Chase and throughout the Temple University Health System.”
With AlphaFold2, Dunbrack says they can now predict the structures of medium-sized proteins to near experimental accuracy in an hour – experiments that might take many months or years to complete. For very large proteins (more than 1200 amino acids), AlphaFold2 often crashes, but Dunbrack’s team implemented the program in a way that can handle them. One such computation was a structure prediction of BRCA2, a protein of more than 3000 amino acids that is a central player in DNA repair and is linked to several types of cancer, including breast and pancreatic. Previous structure predictions were based on the experimental structure of the mouse protein, which tends to overlap with the human protein structure at about 70%. With AlphaFold2, in three days Dunbrack’s team had a much more accurate model that will help guide research around inherited breast cancer mutations.
“Now we can look at that structure and figure out answers to: Where is the mutation? Why is it causing a disruption in the function of this protein?” Dunbrack says. “Potentially, small molecule drugs could be developed to overcome that mutation, for instance, by stabilizing the protein… so you’ve overcome the mutation that somebody may have inherited.”
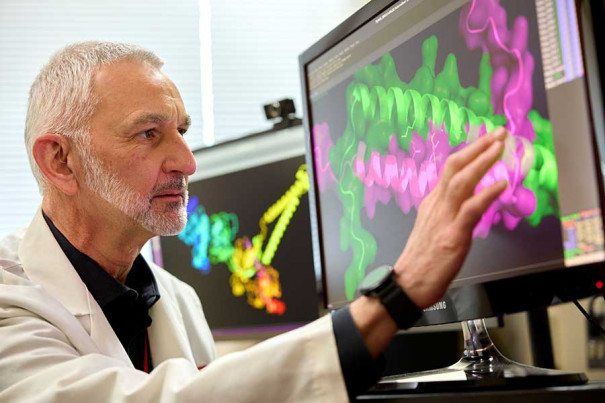
Courtesy of Fox Chase Cancer Center
While the facility was doing protein predictions for Fox Chase before AlphaFold, Mark Andrake, PhD, the manager of the Molecular Modeling Facility, says it’s hard to overstate the difference it has made. In the early 2000s, as few as a third of the models that researchers created of protein structures were totally accurate. By around 2016, he says, we might have improved predictions to about 60%, but those models often took weeks to carefully complete. Such modeling usually required there being a template — an experimental structure of a protein related to the target. With the new AI, they’ve taken a huge leap forward. Now, they’re able to predict accurate models more than 90% of the time, without the former requirement of a template — and with much quicker turnarounds.
“Essentially, instead of the crystal ball of protein structure prediction being hazy and often plagued with things that were inaccurate about a particular model, now we can rely on the AlphaFold models much more,” Andrake says. “It’s making it a lot more feasible to specifically target key proteins in cancer pathways. Recently we were able to help physicians and researchers analyze many mutations found in kidney cancer tumors and help understand the pathogenic role of those variant proteins to disrupt normal cell replication.”
This is a paid partnership between Fox Chase Cancer Center and Philadelphia Magazine