How Researchers Are Using AI to Improve Thyroid Cancer Diagnosis
A new study by Jefferson researchers used artificial intelligence on images of thyroid growths to predict the risk of cancer.
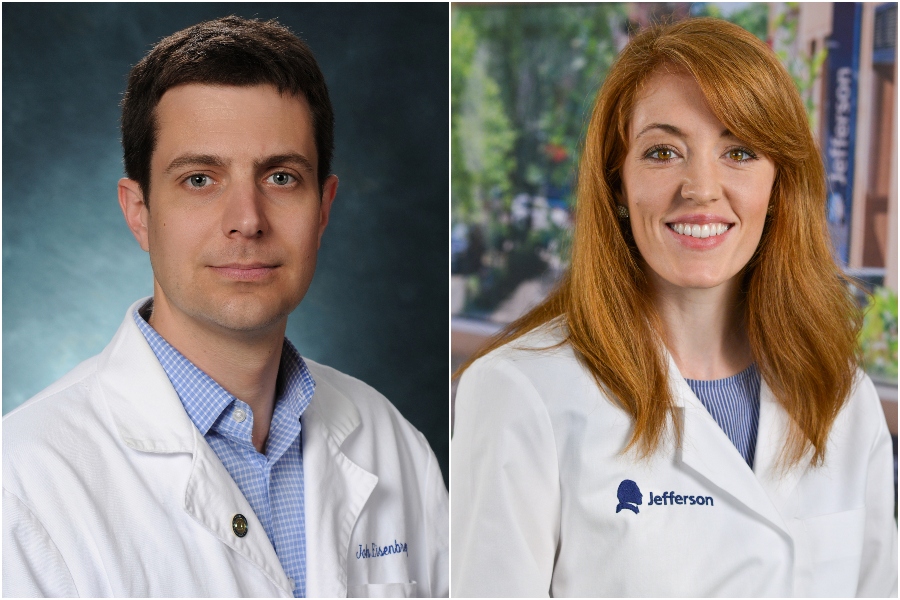
John Eisenbrey, PhD, an associate professor of radiology, and Elizabeth Cottril, MD, an otolaryngologist at Thomas Jefferson University.
Artificial Intelligence (AI) is being used for everything from planting crops to flying planes, and now, it’s being used to diagnose disease. Recent studies show AI is not only capable but possibly better than humans at detecting signs of disease, enabling faster, more accurate diagnoses.
Researchers at Thomas Jefferson University are the latest to investigate the power of machine learning in medicine. A team of Jefferson researchers led by John Eisenbrey, an associate professor of radiology, and Elizabeth Cottril, an otolaryngologist at Thomas Jefferson University, recently conducted a study to see if a non-invasive method of ultrasound imaging, combined with a Google-platform machine-learning algorithm, could be used as a quick and inexpensive first screen for thyroid cancer.
The thyroid is a small gland in the front of the human neck that controls metabolism. Every year, about 12,000 men and 35,000 women get thyroid cancer, and more than 900 men and 1,100 women die from the disease, according to the CDC.
Thyroid cancer begins in the thyroid gland and is typically identified by doctors through ultrasounds or other imaging tools. But thyroid nodules, small lumps that form in the thyroid gland, aren’t always cancerous. In fact, according to Cottril, a majority of thyroid nodules are noncancerous and cause no symptoms. However, because there are currently limited guidelines that tell doctors what to do with thyroid nodules that fall in the gray area (lumps that cannot be easily identified as benign or cancerous) patients are often improperly diagnosed and may undergo unnecessary surgery when the risk of cancer is uncertain.
The Jefferson team developed an algorithm that achieved 97 percent accuracy in identifying benign thyroid growths and 90 percent accuracy in detecting cancerous thyroid growths. The overall accuracy of the algorithm was over 77 percent.
Though the results hint at the promise of expanded use of AI in medicine, will machines ever truly replace a human’s role in diagnosing disease? NextHealth PHL spoke to Eisenbrey and Cottril about the role of AI in medicine and how the tech might be used in the future to improve the diagnosis of disease.
NextHealth PHL: How is thyroid cancer currently diagnosed and is it more difficult to diagnose than other cancers?
Cottril: It is. We usually begin by getting an imaging study done, either an ultrasound, CT or MRI scan. A mass may be further evaluated with that imaging first to confirm that the lump exists and confirm what it looks like in the neck or what it’s connected to or what it is sitting in. The next step is usually to do a biopsy with a needle. This allows us to get a very small sample rather than getting a big chunk of the tissue. That sample is then smeared on to a slide and given to a pathologist who will tell us what the cells indicate.
We have a couple of indicators that we look for in the actual imaging itself that tell us what looks more suspicious for cancer versus what is more likely to be benign. The ultrasound also helps us determine when we need to do a biopsy. When we do decide that it’s necessary to do a biopsy, the sample that we get is often indeterminate. So, it doesn’t mean that it’s not diagnostic. It just means that the cells fall somewhere in between benign and malignant. In other words, they are atypical.
We have a lot of uncertainty when diagnosing thyroid cancer that we use a lot of protocols to get around. It would be amazing to have a more accurate, upfront diagnostic modality.
How often is thyroid cancer misdiagnosed?
Cottril: Without some of the more specific testing that we have now, we were doing a lot of thyroid surgery for these indeterminate nodules maybe five or 10 years ago. If we can make a clearer delineation about what is cancer or what is not cancer, we can save a lot of people from having to go to surgery for what ends up being a benign nodule. We don’t want to do surgery on patients that don’t really need it.
How did your team use AI to improve this process?
Eisenbrey: On the clinical side, we had a large database of ultrasound images from patients that were getting them done as part of the standard of care. On the processing side, a lot of these AI platforms are now commercially available, and the one we used was provided by Google.
We’re a team of scientists, not coders, and we’re not trying to compete with Google. But what we do have is very good clinical expertise in what some of these AI platforms can be used for. And we have very good access to reliable, high-quality imaging from patients. So, with approval from the ethics board, we were able to export all the ultrasound images and upload them into this AI platform.
The Google platform uses a technique called transfer learning that uses architecture from prior projects and customizes it for this clinical application. We spend about 90 percent of the time prepping these images for processing, which builds the actual model. Once the model is created, we can then validate on a subset of patients and see how well it can predict cancer risk.
Your AI algorithm achieved 77 percent accuracy overall. Can the algorithm be improved to get closer to 100 percent accuracy?
Eisenbrey: I think there’s still room for improvement in the overall accuracy. One way is to get more data and work with others to collaborate and create a multi-center repository of these samples. With any of these AI programs, the more data you can put into them, the more accurate your model is going to become.
Will algorithms and machines ever replace humans in the process of diagnosing disease?
Eisenbrey: Maybe sometime in the future but not any time soon. I think that there are a lot of examples out there of AI gone wrong when it’s over-leveraged. At this point, there’s still going to always have to be some type of human supervision. We can use AI to guide physicians to some extent or improve the efficiency of workflows, but in terms of replacing humans, the problems are too complex for that to happen anytime within the next decade.